- Home
- Impact Highlights
- Securing the Energy Network of the Future
Securing the Energy Network of the Future
Electricity and network operators are mandated to prepare the electricity distribution and supply network for the integration of low carbon technologies from a rapid rollout of electric vehicles to embedded renewable generation. The quality of supply to end-use demand relies on accurate active asset management, accurately predicting energy demand, and anticipating any outages which may arise. In response to this challenge, ERPE researchers initiated a collaboration with Scottish Power Energy Networks, one of the ‘big 6’ energy companies in the UK to develop the Networks Constraints Early Warning System (SPEN). Through the research of this project, ERPE was able to use the latest advances in Machine Learning and computer deep learning to enable the SPEN network to accurately manage the existing asset base (cables) and give them increased knowledge and security in upgrading their network for supply and demand of renewable energy.
This innovative technology designed and modelled by ERPE researchers has enabled SPEN to pave the way to decentralised energy networks for future low carbon renewable integration. It has given the sector an understanding of the impact of power consumption in real-time. The simulations and modelling from machine learning provide critical network information to allow detection of areas prone to poor supply quality and voltage violations while protecting consumers privacy. It can also predict scenarios for new investments such as electrical vehicle charging stations and the rollout of smart meters. It is an invaluable tool for the company’s commitment to transitioning to renewable energy, bringing quality assurance and scalability to data analysis in electrical work.
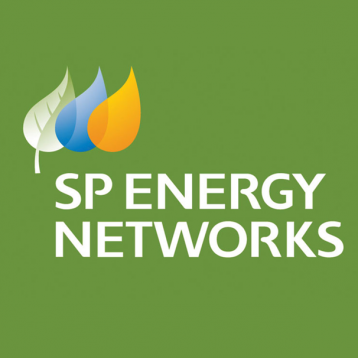
Scottish Power Energy Networks